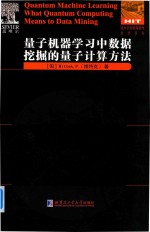
- 作 者:(匈)维特克(P·Wittek)著
- 出 版 社:哈尔滨:哈尔滨工业大学出版社
- 出版年份:2016
- ISBN:7560357591
- 注意:在使用云解压之前,请认真核对实际PDF页数与内容!
在线云解压
价格(点数)
购买连接
说明
转为PDF格式
8
(在线云解压服务)
云解压服务说明
1、本站所有的云解压默认都是转为PDF格式,该格式图书只能阅读和打印,不能再次编辑。
云解压下载及付费说明
1、所有的电子图书云解压均转换为PDF格式,支持电脑、手机、平板等各类电子设备阅读;可以任意拷贝文件到不同的阅读设备里进行阅读。
2、云解压在提交订单后一般半小时内处理完成,最晚48小时内处理完成。(非工作日购买会延迟)
Part One Fundamental Concepts 1
1 Introduction 3
1.1 Learning Theory and Data Mining 5
1.2 Why Quantum Computers? 6
1.3 AHeterogeneous Model 7
1.4 An Overview of Quantum Machine Learning Algorithms 7
1.5 Quantum-Like Learning on Classical Computers 9
2 Machine Learning 11
2.1 Data-Driven Models 12
2.2 Feature Space 12
2.3 Supervised and Unsupervised Learning 15
2.4 Generalization Performance 18
2.5 Model Complexity 20
2.6 Ensembles 22
2.7 Data Dependencies and Computational Complexity 23
3 Quantum Mechanics 25
3.1 States and Superposition 26
3.2 Density Matrix Representation and Mixed States 27
3.3 Composite Systems and Entanglement 29
3.4 Evolution 32
3.5 Measurement 34
3.6 Uncertainty Relations 36
3.7 Tunneling 37
3.8 Adiabatic Theorem 37
3.9 No-Cloning Theorem 38
4 Quantum Computing 41
4.1 Qubits and the Bloch Sphere 41
4.2 Quantum Circuits 44
4.3 Adiabatic Quantum Computing 48
4.4 Quantum Parallelism 49
4.5 Grover's Algorithm 49
4.6 Complexity Classes 51
4.7 Quantum Information Theory 52
Part Two Classical Learning Algorithms 55
5 Unsupervised Learning 57
5.1 Principal Component Analysis 57
5.2 Manifold Embedding 58
5.3 K-Means and K-Medians Clustering 59
5.4 Hierarchical Clustering 60
5.5 Density-Based Clustering 61
6 Pattern Recognition and Neural Networks 63
6.1 The Perceptron 63
6.2 Hopfield Networks 65
6.3 Feedforward Networks 67
6.4 DeepLearning 69
6.5 Computational Complexity 70
7 Supervised Learning and Support Vector Machines 73
7.1 K-Nearest Neighbors 74
7.2 Optimal Margin Classifiers 74
7.3 Soft Margins 76
7.4 Nonlinearity and Kernel Functions 77
7.5 Least-Squares Formulation 80
7.6 Generalization Performance 81
7.7 Multiclass Problems 81
7.8 Loss Functions 83
7.9 Computational Complexity 83
8 Regression Analysis 85
8.1 LinearLeast Squares 85
8.2 Nonlinear Regression 86
8.3 Nonparametric Regression 87
8.4 Computational Complexity 87
9 Boosting 89
9.1 Weak Classifers 89
9.2 AdaBoost 90
9.3 A Family of Convex Boosters 92
9.4 Nonconvex Loss Functions 94
Part Three Quantum Computing and Machine Learning 97
10 Clustering Structure and Quantum Computing 99
10.1 Quantum Random Access Memory 99
10.2 Calculating Dot Products 100
10.3 Quantum Principal Component Analysis 102
10.4 Toward Quantum Manifold Embedding 104
10.5 QuantumK-Means 104
10.6 Quantum K-Medians 105
10.7 Quantum Hierarchical Clustering 106
10.8 Computational Complexity 107
11 Quantum Pattern Recognition 109
11.1 Quantum Associative Memory 109
11.2 The Quantum Perceptron 114
11.3 QuantumNeural Networks 115
11.4 Physical Realizations 116
11.5 Computational Complexity 118
12 Quantum Classification 119
12.1 Nearest Neighbors 119
12.2 Support Vector Machines with Grover's Search 121
12.3 Support Vector Machines with Exponential Speedup 122
12.4 Computational Complexity 123
13 Quantum Process Tomography and Regression 125
13.1 Channel-State Duality 126
13.2 Quantum Process Tomography 127
13.3 Groups,Compact Lie Groups,and the Unitary Group 128
13.4 Representation Theory 130
13.5 Parallel Application and Storage of the Unitary 133
13.6 Optimal State for Learning 134
13.7 Applying the Unitary and Finding the Parameter for the Input State 136
14 Boosting and Adiabatic Quantum Computing 139
14.1 Quantum Annealing 140
14.2 Quadratic Unconstrained Binary Optimization 141
14.3 Ising Model 142
14.4 QBoost 143
14.5 Nonconvexity 143
14.6 Sparsity,Bit Depth,and Generalization Performance 145
14.7 Mapping to Hardware 147
14.8 Computational Complexity 151
Bibliography 153